As we've explored in our previous discussions, Synthetic Data has emerged as a transformative tool in Real-Time Market Research (RTMR), offering unparalleled benefits in:
- Enhanced Privacy: Protecting sensitive information while maintaining data utility
- Increased Speed: Rapidly generating data to meet the demands of real-time insights
- Overcoming Data Gaps: Simulating rare events, forecasting trends, or representing underreported demographics
However, as synthetic data becomes increasingly integral to RTMR workflows, a critical aspect comes into focus: Ethics. The responsible use of synthetic data is not merely a moral imperative, but a strategic one, influencing research integrity, stakeholder trust, and ultimately, business success.
The Importance of Ethical Considerations
- Research Integrity: Ensuring that synthetic data is generated, used, and interpreted in a manner that maintains the validity and reliability of research findings.
- Protection of Sensitive Information: Safeguarding personal, proprietary, or confidential data from potential misuse or exposure.
- Fostering Trust: Transparency and accountability in synthetic data practices are crucial for building and maintaining trust among stakeholders, including customers, partners, and regulatory bodies.
Ethical clarity in the use of synthetic data is crucial for maintaining research integrity, protecting sensitive information, and fostering trust in RTMR findings.
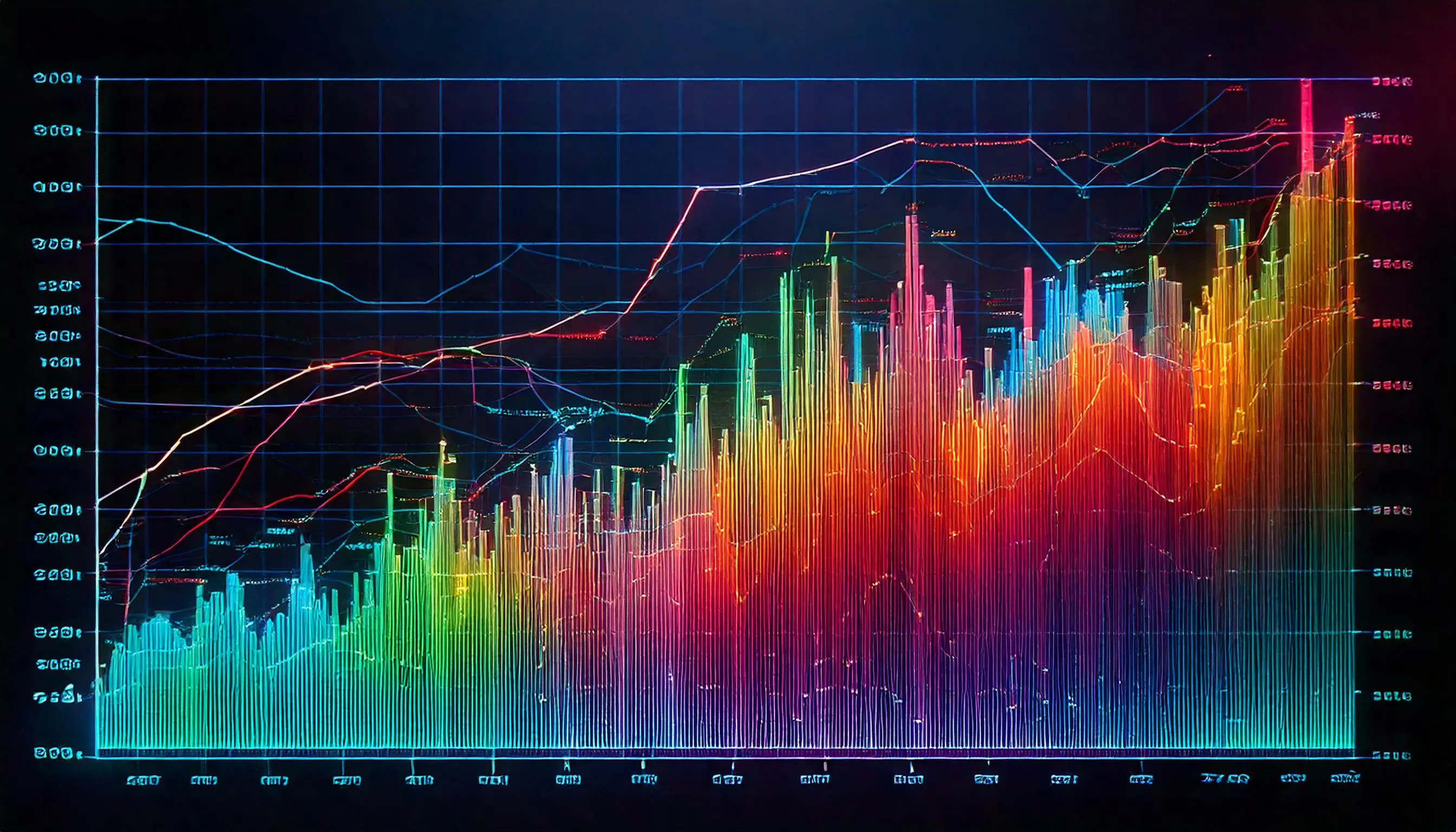
The Challenge: Balancing Data Utility with Individual Privacy in RTMR
In Real-Time Market Research (RTMR), accessing and analyzing sensitive customer data is crucial for informed decision-making. However, this necessity often collides with the imperative to protect individual privacy, particularly in the wake of stringent regulations like the General Data Protection Regulation (GDPR) and the California Consumer Privacy Act (CCPA).
- The Conundrum:
- Data Utility: RTMR requires detailed, accurate data to derive meaningful insights.
- Individual Privacy: Protecting sensitive information from unauthorized access or misuse is paramount.
Synthetic Data to the Rescue:
Synthetic data offers a innovative solution to this challenge, enabling organizations to:
- Maintain Data Utility: Synthetic data retains the statistical properties and value of original data.
- Ensure Privacy: Synthetic data is generated to protect sensitive information, adhering to privacy regulations.
Techniques for Protecting Sensitive Information:
a. Anonymization and Pseudonymization:
- Anonymization: Irreversibly transforming personal data to prevent re-identification.
- Pseudonymization: Replacing identifiable data with artificial identifiers, allowing for re-identification only under authorized conditions.
How it Works in Synthetic Data Generation:
- Data Input: Sensitive customer data (e.g., names, addresses, IDs)
- Anonymization/Pseudonymization: Applying algorithms to transform input data
- Synthetic Data Output: Anonymized or pseudonymized data, retaining statistical value
b. Data Masking and Encryption:
- Data Masking: Concealing sensitive information by replacing it with fictional but realistic data.
- Encryption: Protecting synthetic data during storage and transmission using cryptographic techniques.
Enhanced Security Measures:
- Tokenization: Replacing sensitive data with tokens, usable only in specific contexts.
- Access Controls: Implementing strict permissions to limit synthetic data access.
The Pitfall of Bias: Perpetuating or Introducing Biases in Synthetic Data
Synthetic data, while offering numerous benefits, can also perpetuate or introduce biases if not carefully managed. This can lead to:
- Skewed Insights: Biased synthetic data can result in inaccurate market research findings, influencing misguided strategic decisions.
- Unfair Outcomes: Biases in synthetic data can perpetuate existing social inequalities, particularly in applications involving demographic or personal data.
Recognizing Biases:
Identifying biases is the first step towards mitigation. Two primary sources of bias in synthetic data generation are:
1. Data Source Bias:
- Understanding the Limitations: Recognize the biases and limitations of the original data used for synthesis.
- Common Issues:
- Sampling Bias: If the original data sample is not representative of the population.
- Non-Response Bias: If certain groups are underrepresented due to non-response.
- Measurement Bias: Errors in data collection instruments or procedures.
2. Algorithmic Bias:
- Identifying Potential Biases: Examine the synthetic data generation algorithms for potential biases.
- Common Issues:
- Programming Bias: Biases introduced by the developers' assumptions or preferences.
- Data-Driven Bias: Biases present in the training data that are learned and amplified by the algorithm.
Mitigation Strategies:
1. Diverse and Representative Source Data:
- Ensuring Diversity: Strive for source data that is diverse and representative of the population.
- Data Validation: Regularly validate the source data to detect and address any biases.
2. Regular Audits and Testing:
- Continuous Monitoring: Regularly audit synthetic data for biases and test for fairness.
- Algorithmic Adjustments: Adjust the synthetic data generation algorithms based on audit findings to mitigate biases.
Best Practice: Implementing a Bias Impact Assessment Framework
- Framework Components:
- Bias Identification: Systematically identify potential biases in source data and algorithms.
- Risk Assessment: Evaluate the impact of identified biases on synthetic data quality and fairness.
- Mitigation Strategies: Develop and implement strategies to address identified biases.
- Continuous Monitoring: Regularly review and update the framework to ensure ongoing fairness and accuracy.
The Importance of Transparency: Building Trust with Stakeholders
In the context of Real-Time Market Research (RTMR), transparency is paramount when utilizing synthetic data. Openly disclosing the use of synthetic data is essential for:
- Building Trust: With stakeholders, including customers, partners, and regulatory bodies.
- Maintaining Credibility: Ensuring the integrity of research findings and the organization as a whole.
- Fostering Collaboration: Encouraging open communication and cooperation among stakeholders.
Disclosure Guidelines: Ensuring Clarity and Transparency
To maintain transparency, adhere to the following disclosure guidelines when utilizing synthetic data in RTMR:
1. Clear Labeling: Explicit Disclosure of Synthetic Data Use
- Explicit Indication: Clearly state when research findings, reports, or insights are based on synthetic data.
- Labeling Examples:
- "This market analysis is based on synthetic data generated from anonymized customer transactions."
- "The forecasted trends are derived from synthetic data, simulating potential market scenarios."
2. Methodology Explanation: Providing a Concise Overview
- Process Description: Offer a brief, yet informative, overview of the synthetic data generation process.
- Key Aspects to Cover:
- Data sources used for synthesis
- Synthetic data generation algorithms employed
- Any data transformations or aggregations applied
Example of Methodology Explanation:
"Our synthetic data is generated using a combination of anonymized customer data and machine learning algorithms. The process involves:
- Data Anonymization: Removing identifiable information from customer datasets.
- Synthesis: Utilizing a proprietary algorithm to create synthetic data that mirrors the statistical properties of the original data.
- Quality Check: Verifying the synthetic data's accuracy and consistency with the original data's distributions."
3. Limitations Acknowledgement: Open Discussion of Potential Biases and Limitations
- Transparent Acknowledgement: Openly discuss the potential limitations and biases associated with the synthetic data.
- Aspects to Address:
- Known biases in the synthetic data generation process
- Limitations in the data's ability to fully represent the market or population
- Any assumptions made during the synthesis process
Example of Limitations Acknowledgement:
"While our synthetic data is designed to closely mirror real-world market trends, it is not without limitations. We acknowledge the potential for:
- Algorithmic bias in the synthetic data generation process, which we continuously monitor and address.
- Underrepresentation of certain demographic groups due to limitations in our source data.
- Assumptions regarding market behaviors, which are regularly reviewed and updated based on new insights."
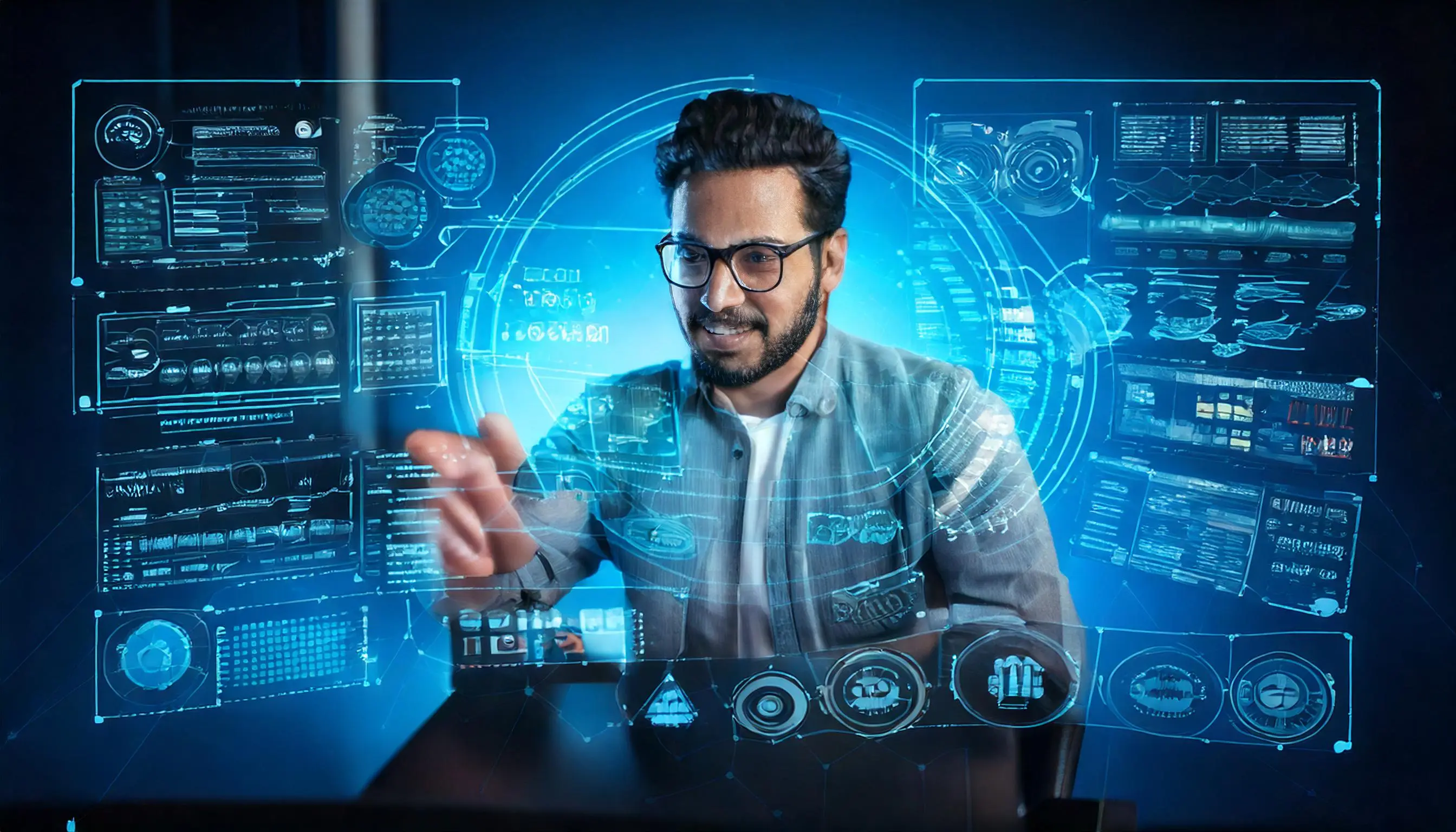
As we conclude our exploration of the ethical considerations surrounding synthetic data in Real-Time Market Research (RTMR), it's clear that responsible innovation is key to unlocking the full potential of this powerful tool. By acknowledging and addressing the challenges of privacy, bias, and transparency, organizations can ensure that their use of synthetic data not only drives business success but also upholds the highest standards of ethics and integrity.